摘要:通过Arrow管理系统,可以高效地管理数据。该系统提供了强大的数据存储和处理能力,支持多种数据类型和大规模数据集的处理。Arrow能够优化数据存储和访问速度,提高数据处理效率。Arrow还提供了易于使用的API和灵活的编程接口,使得开发人员可以轻松地集成和使用该系统,从而更好地管理和分析数据。Arrow是一个可靠的数据管理工具,适用于各种规模的数据处理任务。
在之前的数据挖掘:是时候更新一下TCGA的数据了推文中,保存TCGA的数据就是使用Arrow格式,因为占空间小,读写速度快,多语言支持(我主要使用的3种语言都支持)

Format
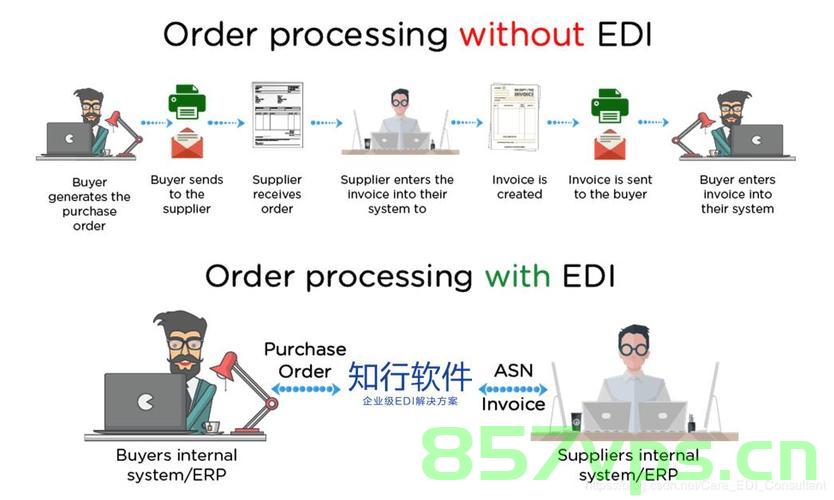
https://arrow.apache.org
Apache Arrow defines a language-independent columnar memory format for flat and hierarchical data, organized for efficient analytic operations on modern hardware like CPUs and GPUs. The Arrow memory format also supports zero-copy reads for lightning-fast data access without serialization overhead.
Language Supported
Arrow's libraries implement the format and provide building blocks for a range of use cases, including high performance analytics. Many popular projects use Arrow to ship columnar data efficiently or as the basis for analytic engines.
Libraries are available for C, C++, C#, Go, Java, JavaScript, Julia, MATLAB, Python, R, Ruby, and Rust.
Ecosystem
Apache Arrow is software created by and for the developer community. We are dedicated to open, kind communication and consensus decisionmaking. Our committers come from a range of organizations and backgrounds, and we welcome all to participate with us.
R
install.packages("arrow")
library(arrow)
# write iris to iris.arrow and compressed by zstd
arrow::write_ipc_file(iris,'iris.arrow', compression = "zstd",compression_level=1)
# read iris.arrow as DataFrame
iris=arrow::read_ipc_file('iris.arrow')
python
# conda install -y pandas pyarrow
import pandas as pd
# read iris.arrow as DataFrame
iris=pd.read_feather('iris.arrow')
# write iris to iris.arrow and compressed by zstd
iris.to_feather('iris.arrow',compression='zstd', compression_level=1)
Julia
using Pkg
Pkg.add(["Arrow","DataFrames"])
using Arrow, DataFrames
# read iris.arrow as DataFrame
iris = Arrow.Table("iris.arrow") |> DataFrame
# write iris to iris.arrow, using 8 threads and compressed by zstd
Arrow.write("iris.arrow",iris,compress=:zstd,ntasks=8)
还没有评论,来说两句吧...